1. Which of the following is/are classification tasks?
a. Find the gender of a person by analyzing his writing style
b. Predict the price of a house based on floor area. number of rooms. etc.
C. Predict whether there will be abnormally heavy rainfall next year
d. Predict the number of conies of a book that will be sold this month
Answer :- a. Find the gender of a person by analyzing his writing style c. Predict whether there will be abnormally heavy rainfall next year Explanation: a. Finding the gender of a person based on writing style involves classifying the person into one of two classes - male or female. c. Predicting whether there will be abnormally heavy rainfall next year involves classifying the occurrence of heavy rainfall as either "abnormally heavy rainfall" or "not abnormally heavy rainfall." This can be treated as a binary classification problem.
2. A feature F1 can take certain values: A, B, C, D, E, F, and represents the grade of students from a college. Which of the following statement is true in the following case?
a. Feature F1 is an example of a nominal variable.
b. Feature F1 is an example of an ordinal variable.
c. It doesn’t belong to any of the above categories.
d. Both of these
Answer :- b. Feature F1 is an example of an ordinal variable. Explanation: In statistics, variables can be classified into different types, and two common types are nominal and ordinal variables. Nominal variables are categorical variables with no inherent order. The categories in a nominal variable cannot be ranked or ordered in any meaningful way. Examples of nominal variables are eye color, country names, or the types of fruits. Ordinal variables, on the other hand, have categories with a natural order or ranking. While the exact differences between the categories may not be well-defined, there is a relative ordering among them. Examples of ordinal variables are educational levels (e.g., high school, undergraduate, graduate) or ratings like "good," "better," and "best." In this case, the feature F1 represents the grades of students from a college, and these grades likely have an inherent order such as A being better than B, and so on. Therefore, F1 is an example of an ordinal variable.
3. Suppose I have 10,000 emails in my mailbox out of which 200 are spams. The spam detection system detects 150 emails as spams, out of which 50 are actually spam. What is the precision and recall of my spam detection system?
a. Precision = 33.333%. Recall = 25%
b. Precision = 25%, Recall = 33.33%
c. Precision = 33.33%, Recall = 75%
d. Precision = 75%, Recall = 33.33%
Answer :- a. Precision = 33.33%. Recall = 25%
4. Which of the following statements describes what is most likely TRUE when the amount of training data increases?
a. Training error usually decreases and generalization error usually increases.
b. Training error usually decreases and generalization error usually decreases.
C. Training error usually increases and generalization error usually decreases.
d. Training error usually increases and generalization error usually increases.
Answer :- a
5. You trained a leaming algorithm, and plot the learning curve. The following figure is obtained.
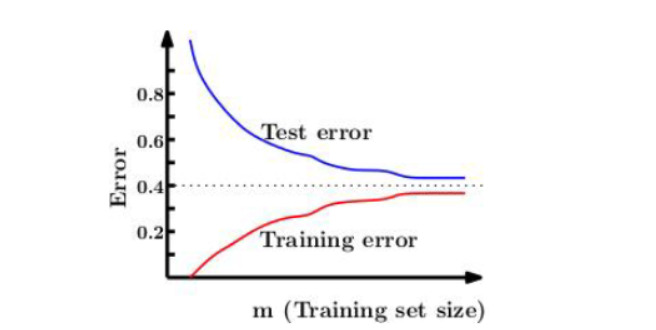
The algorithm is suffering from
a. High bias
b. High variance
c. Neither
Answer :- a
6. I am the marketing consultant of a leading e-commerce website. I have been given a task of making a system that recommends products to users based on their activity on Facebook. I realize that user interests could be highly variable. Hence, I decide to
T1) Cluster the users into communities of like-minded people and
T2) Train separate models for each community to predict which product category (e.g., electronic gadgets. cosmetics. etc.) would be the most relevant to that community.
The task T1 is a/an _________ learning problem and T2 is a/an ________problem.
Choose from the options:
a. Supervised and unsupervised
b. Unsupervised and supervised
c. Supervised and supervised
d. Unsupervised and unsupervised learning problem and I2 is a/an
Answer :- b
7.
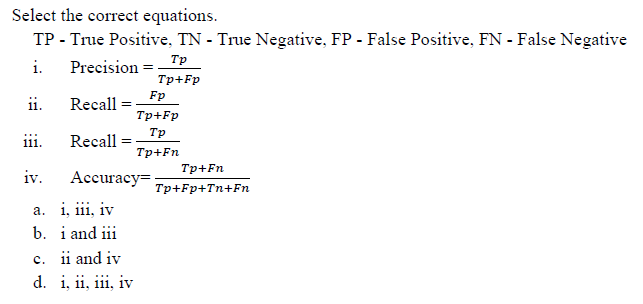
a. i, iii. IV
b. i and iii
c. 11 and iv
d. i. ii, iii. iv
Answer :- a. i, iii. IV
8. Which of the following tasks is NOT a suitable machine learning task(s)?
a. Finding the shortest path between a pair of nodes in a graph
b. Predicting if a stock price will rise or fall
c. Predicting the price of petroleum
d. Grouping mails as spams or non-spams
Answer :- a. Finding the shortest path between a pair of nodes in a graph. Explanation: Machine learning is not typically used for finding the shortest path between nodes in a graph. This problem can be efficiently solved using algorithms like Dijkstra's algorithm or A* search algorithm, which are specifically designed for this purpose and do not involve learning from data.
9. Which of the following is/are associated with overfitting in machine learning?
a. High bias
b. Low bias
c. Low variance
d. High variance
e. Good performance on training data
f. Poor performance on test data
Answer :- b. Low bias d. High variance e. Good performance on training data f. Poor performance on test data Explanation: High variance (option d) refers to a model that is too complex and captures noise or random fluctuations in the training data. As a result, it performs well on the training data but poorly on unseen test data, indicating overfitting. Good performance on training data (option e) is a common characteristic of overfitting. The overfitted model fits the training data closely, leading to high accuracy or low error on the training set. Poor performance on test data (option f) is another sign of overfitting. The overfitted model does not generalize well to new, unseen data, resulting in lower accuracy or higher error on the test set compared to the training set.
10. Which of the following statements about cross-validation in machine learning is/are true?
a. Cross-validation is used to evaluate a model’s performance on the training data.
b. Cross-validation guarantees that a model will generalize well to unseen data.
c. Cross-validation is only applicable to classification problems and not regression problems.
d. Cross-validation helps in estimating the model’s performance on unseen data by simulating the test phase.
Answer :- d
11. What does k-fold cross-validation involve in machine learning?
a. Splitting the dataset into k equal-sized training and test sets.
b. Splitting the dataset into k unequal-sized training and test sets.
c. Partitioning the dataset into k subsets, and iteratively using each subset as a validation set while the remaining k-1 subsets are used for training.
d. Dividing the dataset into k subsets, where each subset represents a unique class label for classification tasks.
Answer :- c
12. What does the term “feature space” refer to in machine learning?
a. The space where the machine learning model is trained.
b. The space where the machine learning model is deployed.
c. The space which is formed by the input variables used in a machine leaming model.
d. The space where the output predictions are made by a machine learning model.
Answer :- c. The space which is formed by the input variables used in a machine learning model. Explanation: In machine learning, the term "feature space" refers to the space formed by the input variables (features) used to train a machine learning model. Each data point in the dataset represents a point in this feature space, where the coordinates are the values of the input features. For example, if you have a dataset with two features, such as "age" and "income," then the feature space would be a two-dimensional space where each data point is represented by a pair of values (age, income). Machine learning algorithms work by trying to find patterns and relationships in this feature space that can be used to make predictions or classifications. The goal is to find a decision boundary or decision surface that separates different classes or groups based on their feature values.
13. Which of the following statements is/are true regarding supervised and unsupervised learning?
a. Supervised learning can handle both labeled and unlabeled data.
b. Unsupervised learning requires human experts to label the data.
c. Supervised learning can be used for regression and classification tasks.
d. Unsupervised learning aims to find hidden patterns in the data.
Answer :- c. Supervised learning can be used for regression and classification tasks. d. Unsupervised learning aims to find hidden patterns in the data.
14. One of the ways to mitigate overfitting is
a. By increasing the model complexity
b. By reducing the amount of training data
c. By adding more features to the model
d. By decreasing the model complexity
Answer :- d. By decreasing the model complexity
15. How many Boolean functions are possible with N features?
a. (22N)
b. (2N)
C. (N2)
d. (4N)
Answer :- a (22N) (Not Sure)